Paddle Blog
Discover what sustainable growth in SaaS really looks like with expert insights shared every month
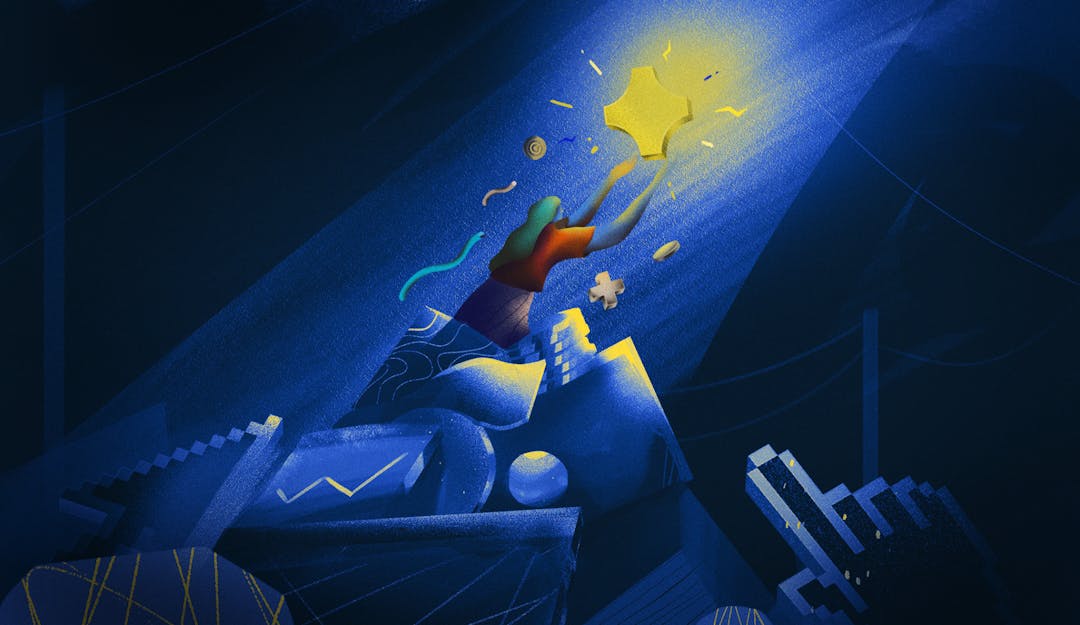
SaaS market report for March 2024: Growth slows for B2B, while B2C hits a 2-year high
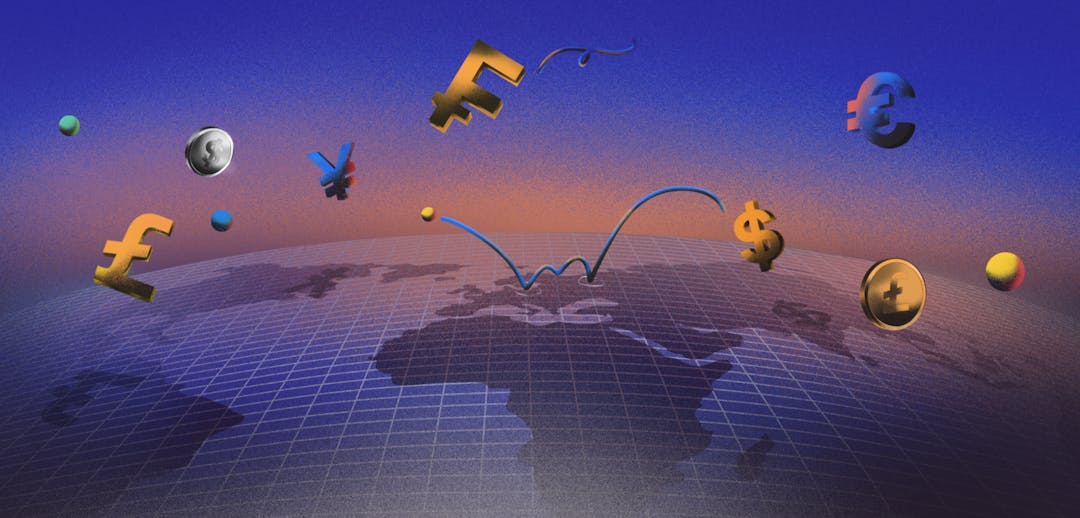
Payment routing part 2: Developing Paddle’s Rules Engine
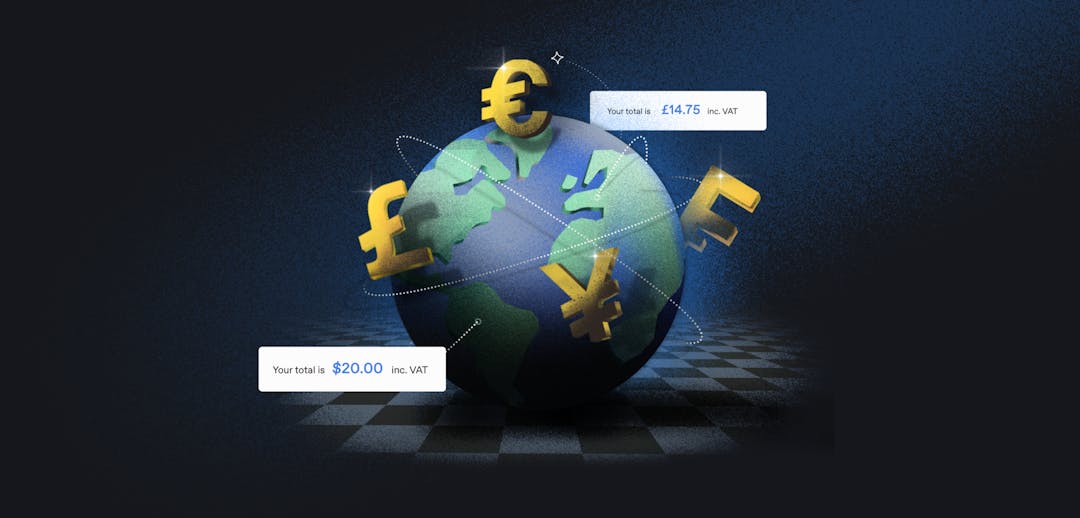
Payment routing part 1: Unifying the payments lifecycle
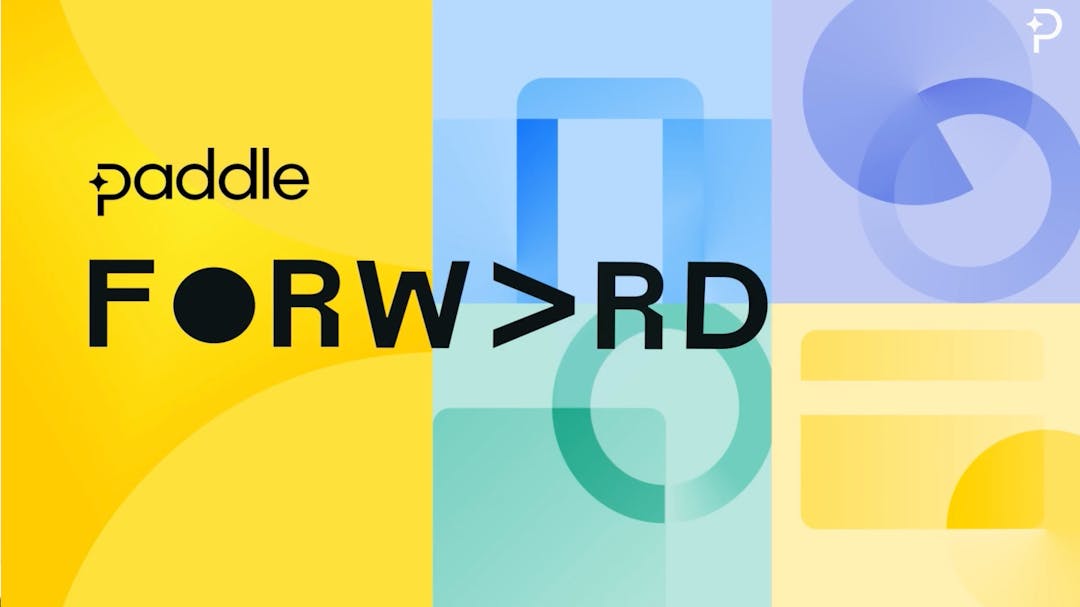
What’s new in Paddle [Q1 2024] - Paddle Forward highlights
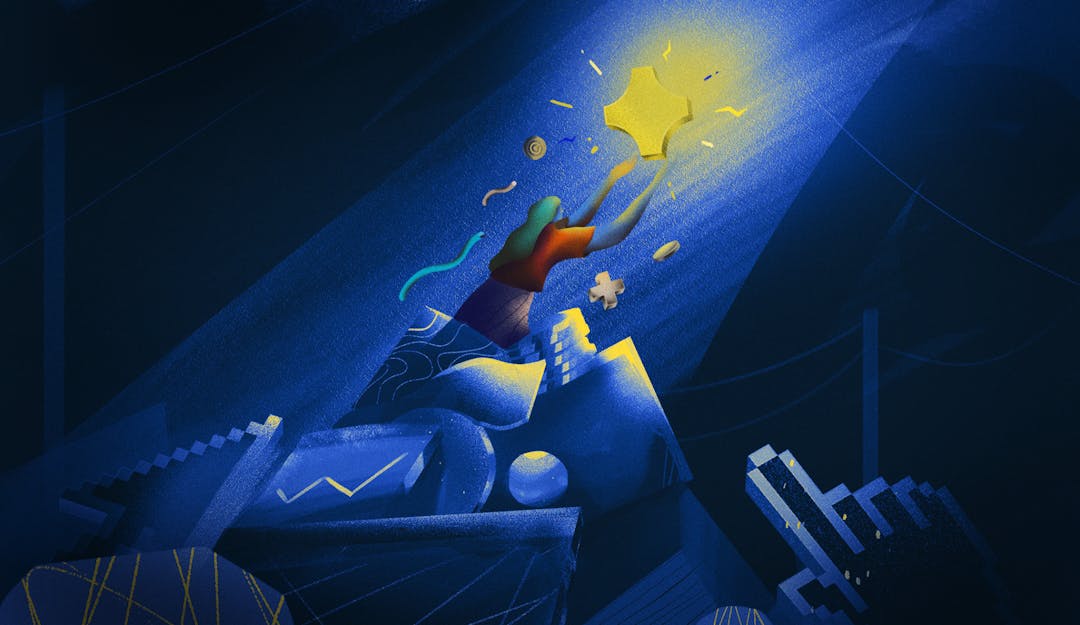
SaaS market report for February 2024: Promising momentum for B2B and B2C
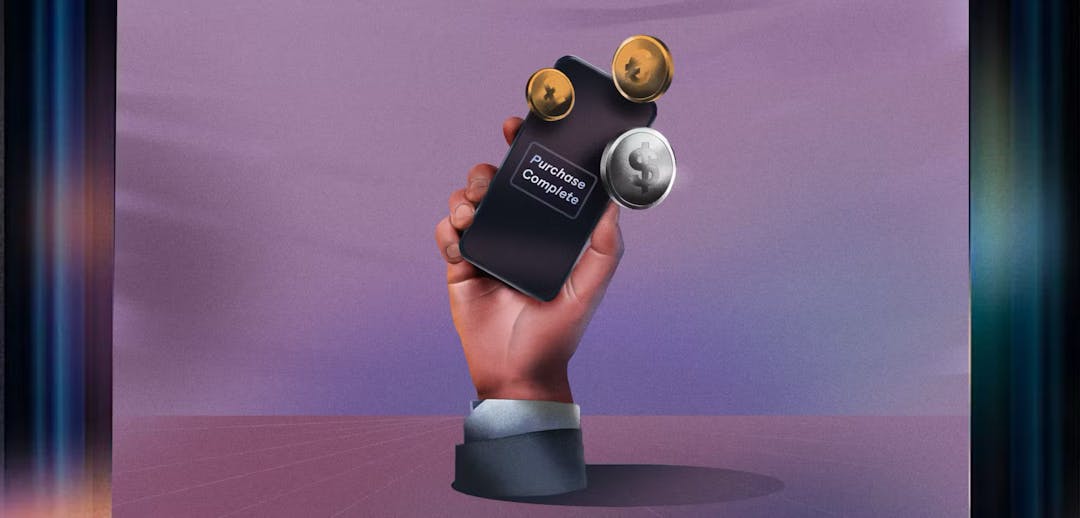
How to unlock revenue growth for your mobile app on the web
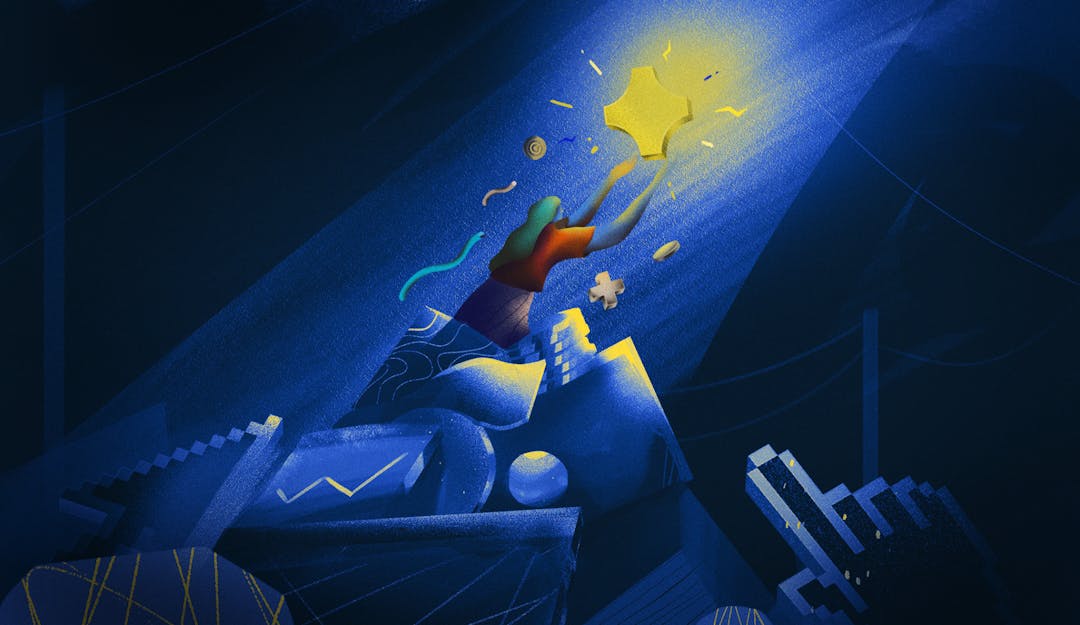
SaaS market report for January 2024: Growth surges back
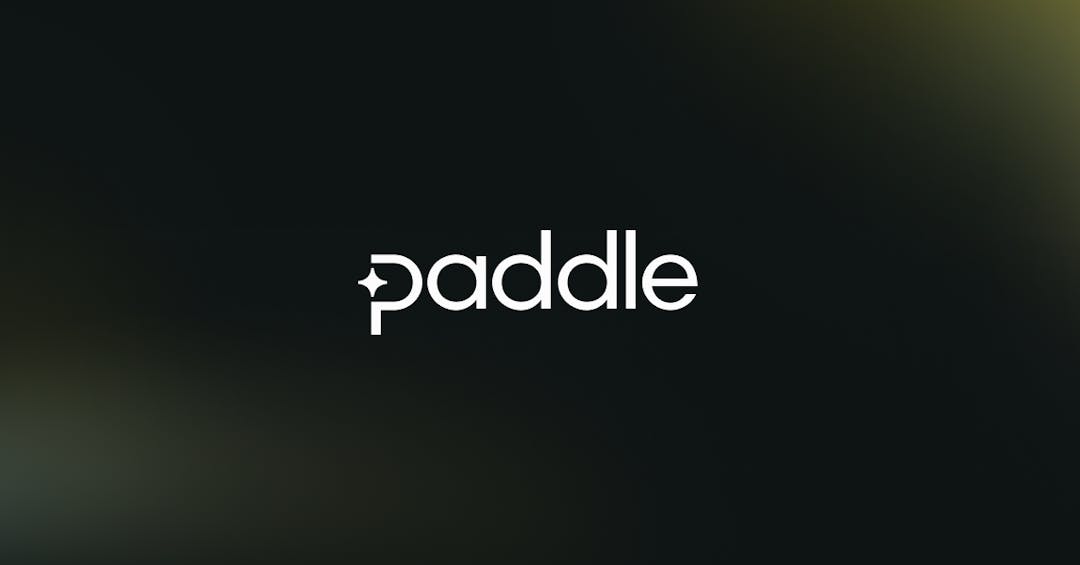
Apple DMA Changes - What this means for in-app purchases
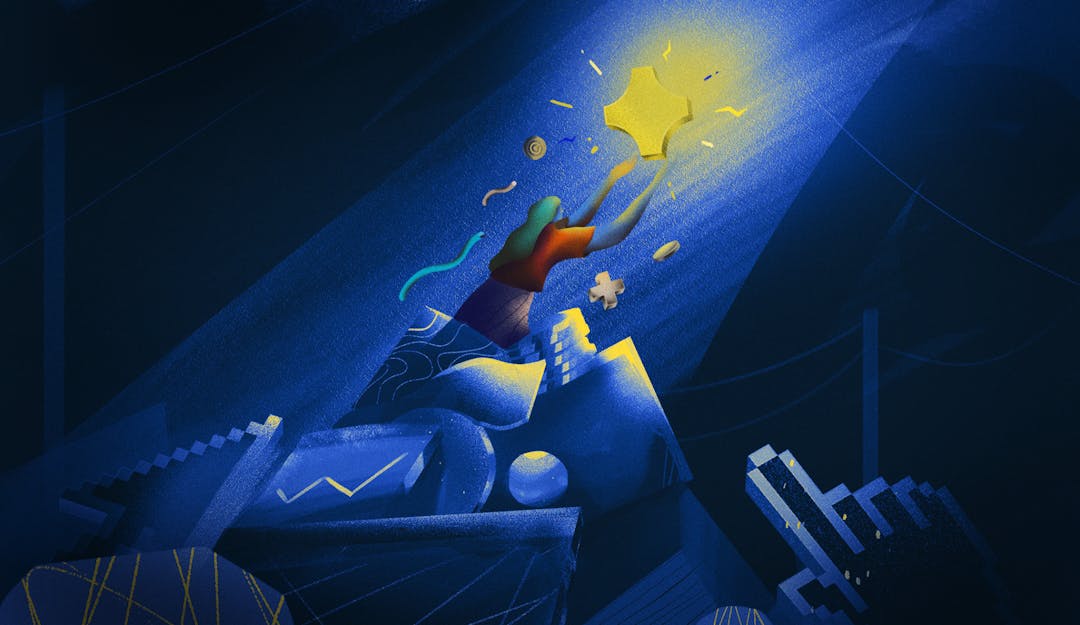
2023 B2B SaaS market report: Is the worst behind us?
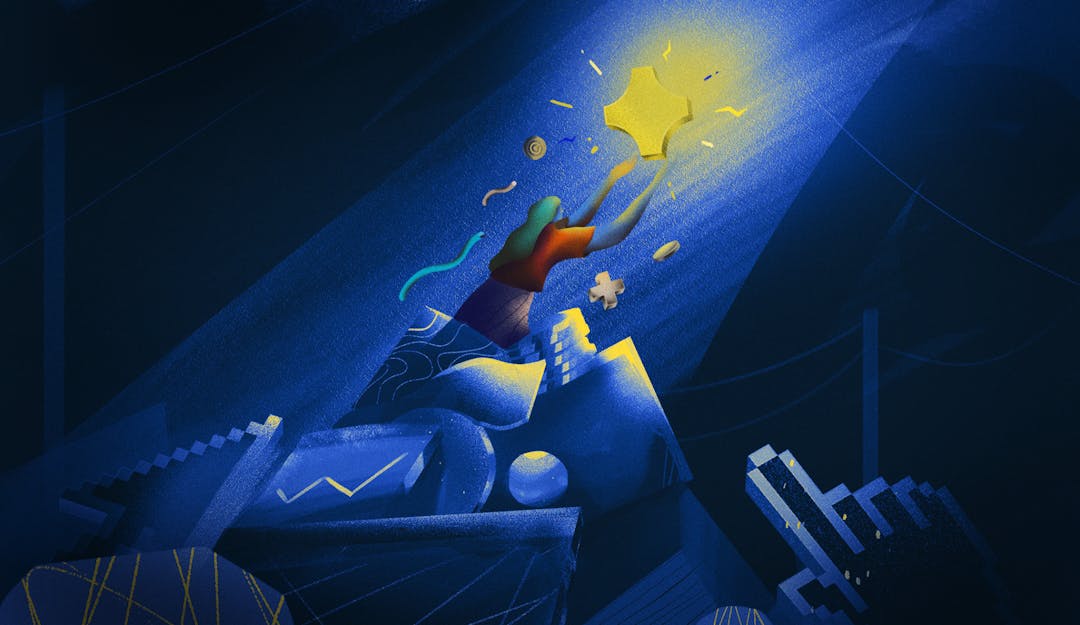
SaaS and subscription market report for November 2023: B2C takes a hit while B2B growth remains slow
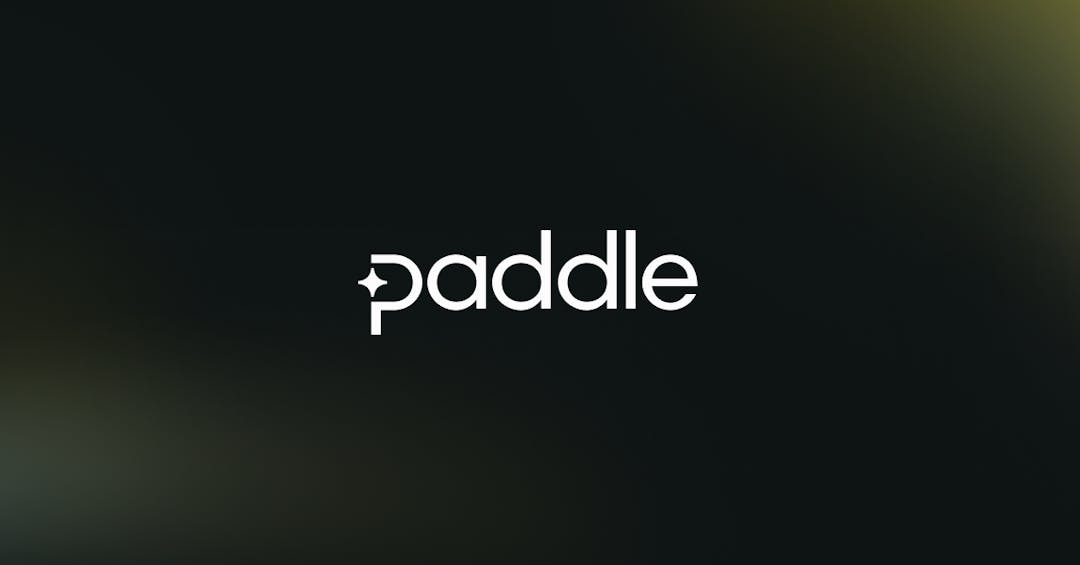
Epic Games win vs Google: The shift from App to Web
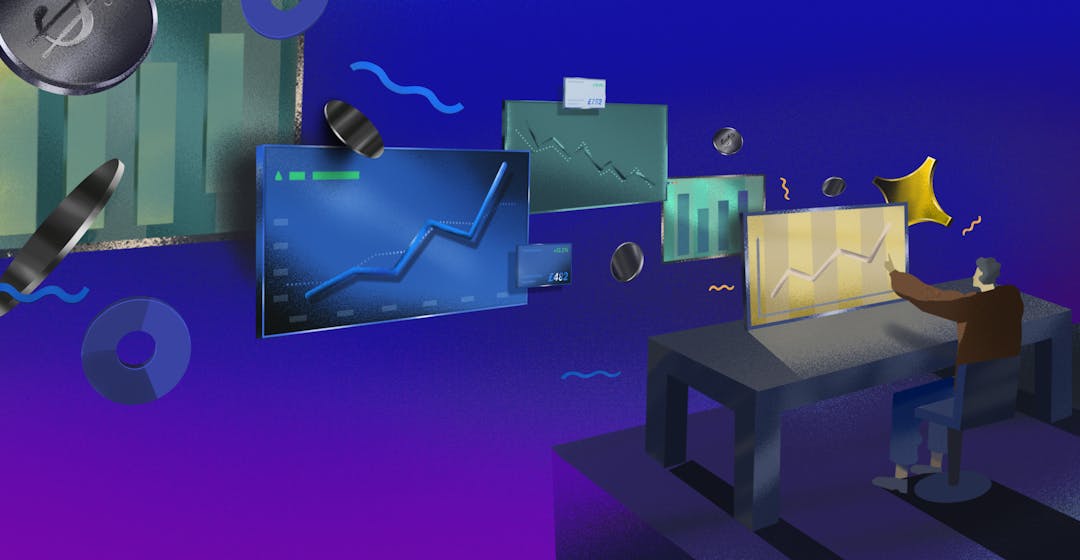
How to become a SaaS outlier in 2024: Accelerating growth with a lean team